The amount of resources needed to train and deploy state of the art deep neural models is enormous, from CPUs, GPUs and storage to bandwidth and dataset acquisition (with possible annotations). More importantly, it steadily increases at an unsustainable pace, leading to a growing and alarming environmental footprint, and results in significant societal tensions. This raises serious concerns, not only in flagship domains such as computer vision (CV) and natural language processing (NLP) where all players become dependent on the main worldwide players (with the risk of an AI oligopoly), but also in many domains where data is scarce and resource-hungry models cannot be successfully trained with current know-how.
The major challenge of the SHARP project is to achieve a leap forward in frugality by designing, analyzing and deploying intrinsically efficient models (neural or not) able to achieve the versatility and performance of the best models while requiring only a vanishing fraction of the resources currently needed.
To achieve its vision, SHARP will design a principled theoretical and algorithmic framework to leverage prior knowledge and the modern avatars of the notion of sparsity of predictors and/or algorithms, and establish a new paradigm of computational representation learning bypassing the current technical and computing bottlenecks. Two showcase demonstrations of the impact of SHARP will be the frugal training of compact transformers with negligible performance loss, and the development of effective representation learning models on small unlabeled datasets, for a selected downstream application. To achieve such frugality in AI, SHARP will rely on three pillars: frugal architectures, frugal learning principles, and learning with small and scarce datasets.
Keywords: Statistical learning, algorithmic efficiency, sparsity, deep learning, computer vision, natural language processing
A project funded by the France 2030 program, managed by the ANR, project ANR-23-PEIA-0008, in the context of the PEPR IA.
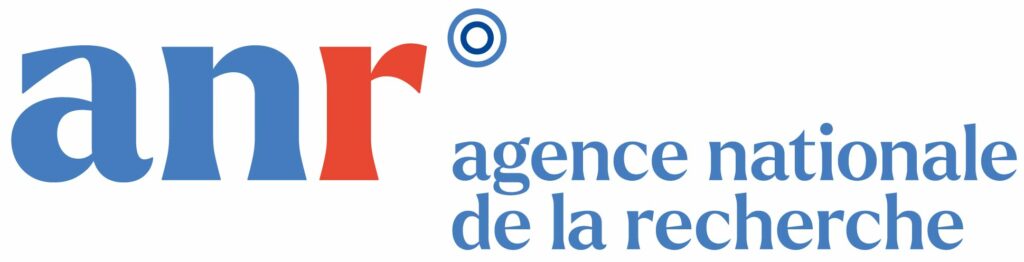
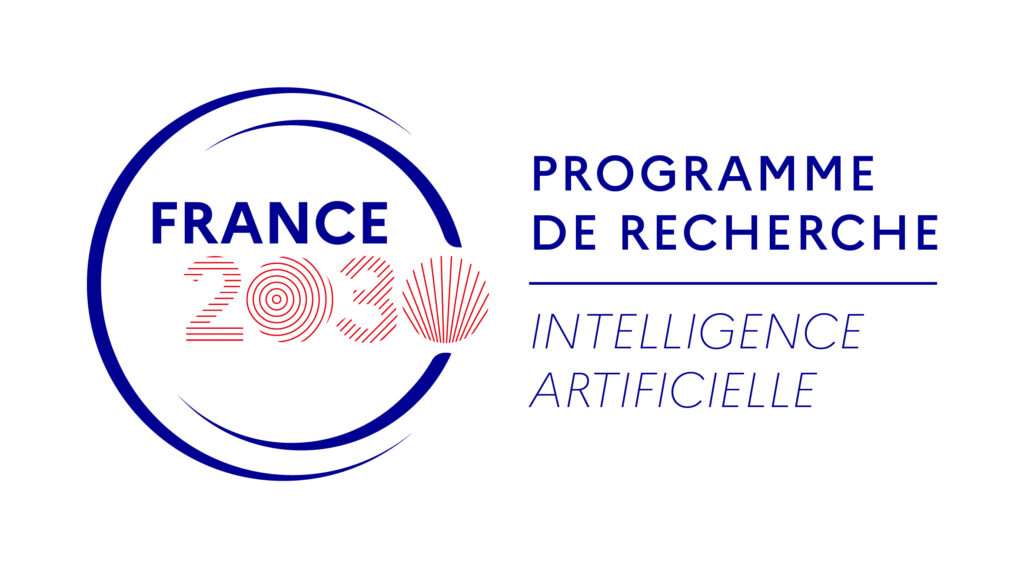