The BADASS project
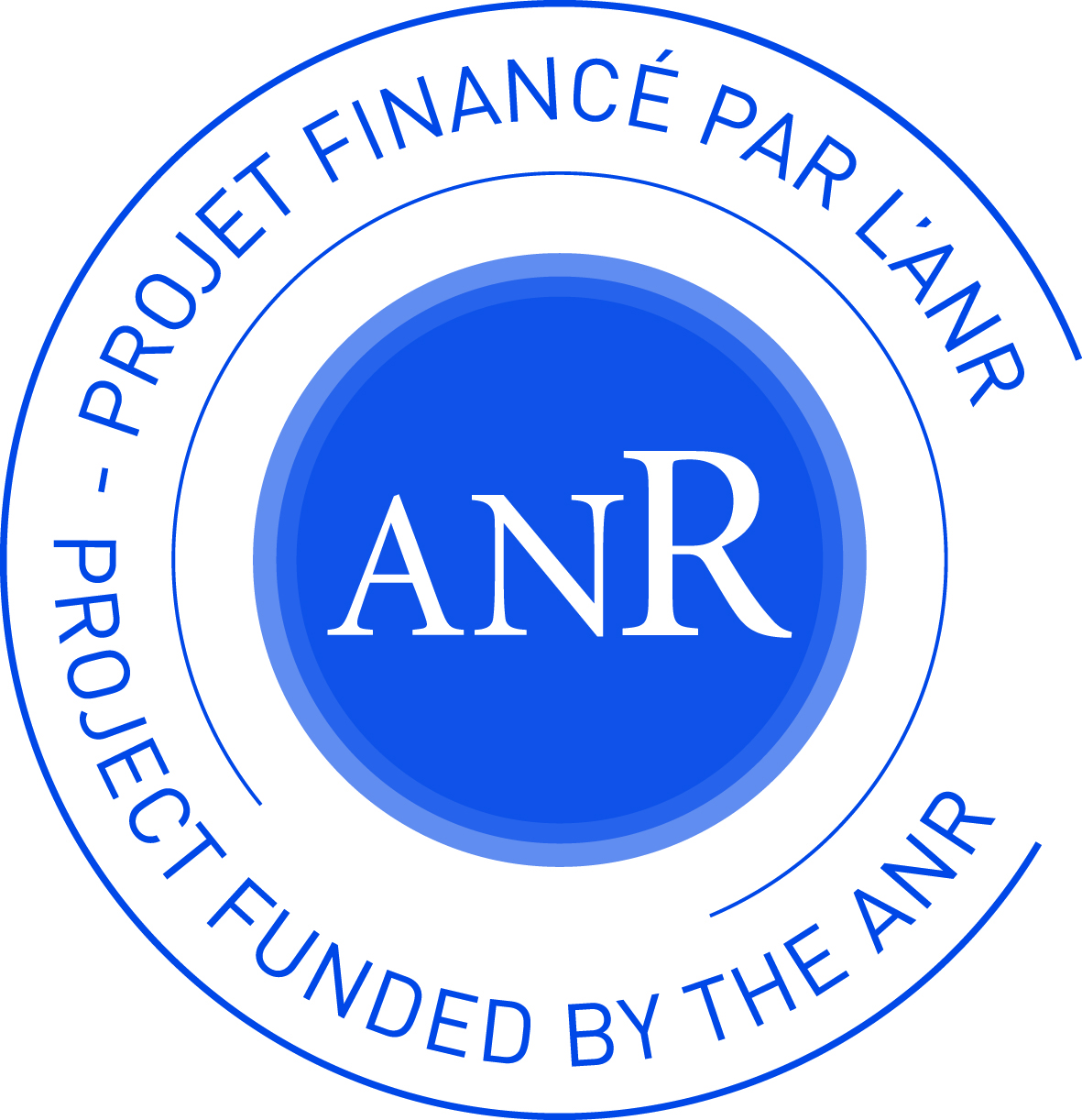
The project "Bandits Against Non-stationarity and Structure" (BADASS) is a JCJC project funded by the French ANR (ANR-16-CE40-0002).
It is headed by Odalric-Ambrym Maillard, together with Emilie Kaufmann and Richard Combes.
We intend to focus on the following objectives:
- To broaden the range of optimal strategies for stationary MABs: current strategies are only known to be provably optimal in a limited range of scenarios for which the class of distribution (structure) is perfectly known; also, recent heuristics possibly adaptive to the class need to be further analyzed.
- To strengthen the literature on pure sequential prediction (focusing on a single arm) for non-stationary signals via the construction of adaptive confidence sets and a novel measure of complexity: traditional approaches consider a worst-case scenario and are thus overly conservative and non-adaptive to simpler signals.
- To embed the low-rank matrix completion and spectral methods in the context of reinforcement learning, and further study models of structured environments: promising heuristics in the context of e.g. contextual MABs or Predictive State Representations require stronger theoretical guarantees.

Visiting researcher: Junpei Komiyama
We are glad that Junpei Komiyama is visiting us from October 01 to November 15.
Pr. Tze Leung Lai at the EWRL
The ANR BADASS funded the visit of Professor Tze Leung Lai as an invited speaker at the European Workshop on Reinforcement Learning, October 01-03, Lille. This is a historical event for the field of multi-armed bandit and reinforcement learning.
Postdoctorant member
We are happy to announce that Mohammad Sadegh Talebi has joined the BADASS project in June 2018.
Hiring of a new Ph.D. student.
We finally complete the hiring process for the Ph.D. student of the BADASS project, after a tough selection procedure involving candidates from all over the world.