Multicenter learning using supervised GAN
Database
In this study, 128 patients with prostate cancer from four datasets (D1, D2, D3 and D4) had CT and MR scans (standard MRI (for D1, D2, and D3) and MRI-Linac (for D4)) in the treatment position. The dataset D1 is composed by 39 patients from one care center, CT scans were acquired with a GE LightSpeedRT large-bore scanner or a Toshiba Aquilion. For MR images, 3D T2-weighted SPACE sequences were acquired on a 3T Siemens Skyra MRI scanner [21]. For the second dataset (D2), the 30 CT scans were acquired on a Philips BigBore and the T2 MRIs on an 1.5T Siemens Skyra MRI scanner. Bladders are injected on the CTs with a contrast agent. The third dataset (D3) is the public GoldAtlas [22] composed of 19 patients from 3 different centers with 1.5T and 3T MRI. Finally, the fourth dataset (D4) is composed of 40 patients, CT were acquired on a GE Light-SpeedRT16 and T2 MRI were acquired on a 0.35T MRIdian (ViewRay) MRI-Linac.

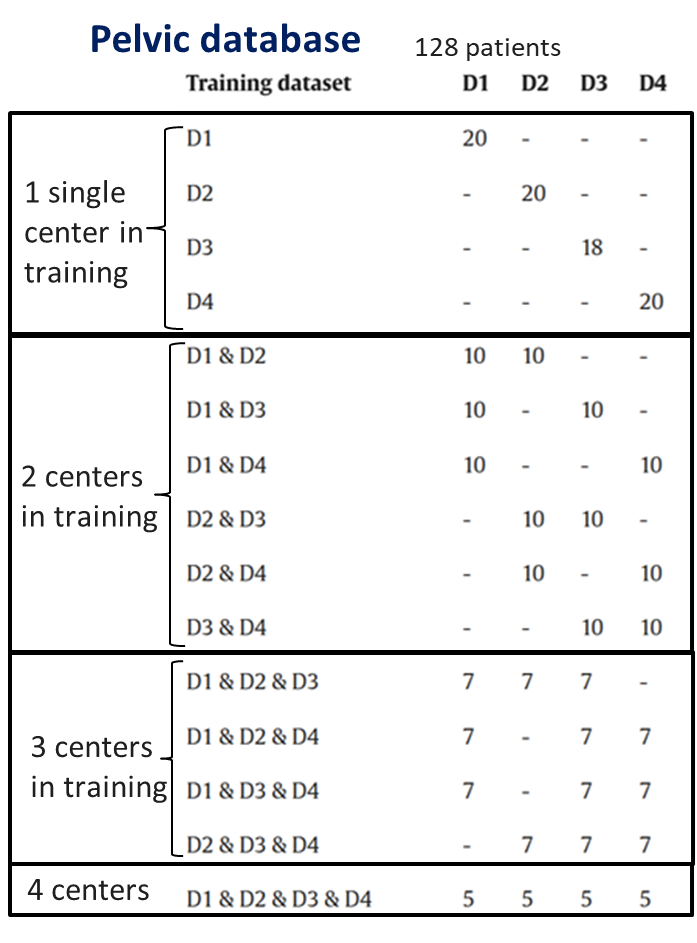
For this study, the 2D Cycle-GAN architecture proposed by Zhu et al. was used in a supervised context.
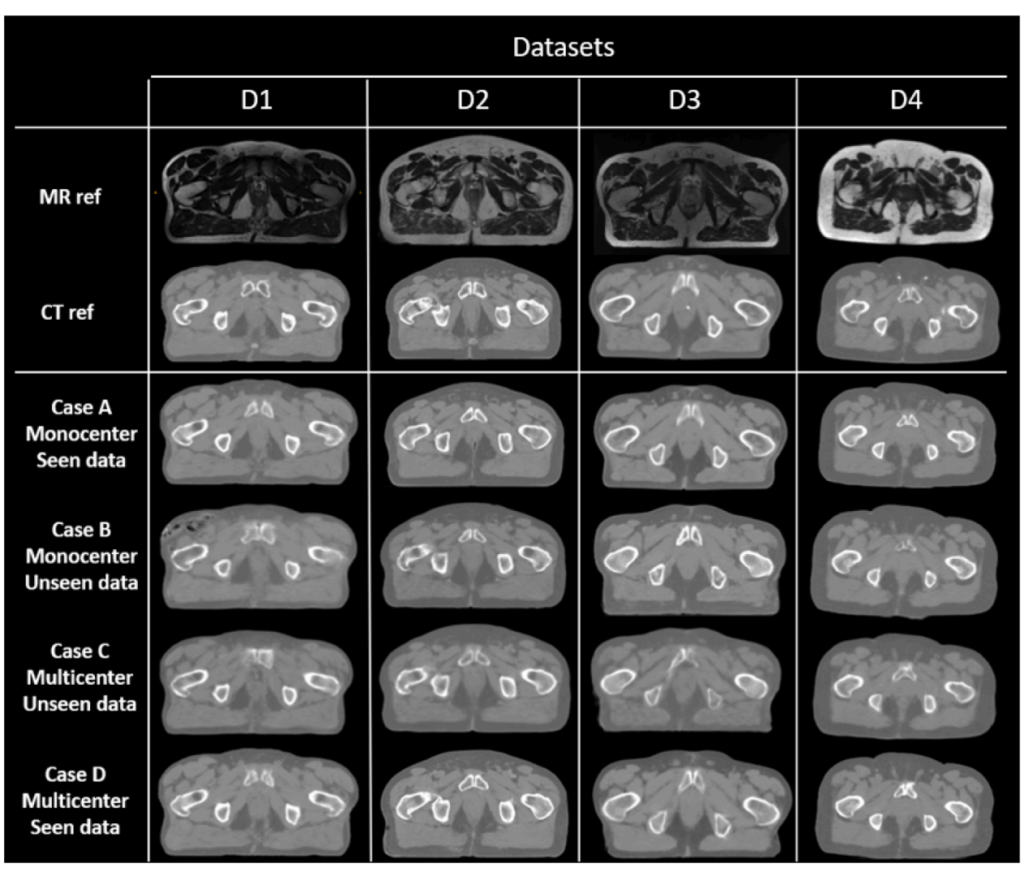