Physical Activity Classification
Challenges
As already mentioned, the SHERPAM system will allow cross applications including monitoring of PA and EE in healthy (athletes) or disabled (HF, stroke, Diabetes, Obesity, peripheral artery diseases…) populations. Methods for monitoring the human physical activity were recently investigated in order to assess the health status of the individuals and thus promote a healthier lifestyle. The challenge here is to propose some relevant signal processing methods in order to recognize the activity without proceeding Features extraction.
At very long term, the objective is to recognizee the activity of the patient in order to better estimate the Energy Expenditure associated.
Achievements
A data collection platform including four accelerometer sensors mounted on the chest, ankle, wrist and hip, is used to record five activities: running, walking, cycling, resting and car riding.The database is constructed with the aim to extract a signal model for each activity based on pertinent indicators that allow a maximum margin separation and compare the qualities of the two body sensor sets in terms of physiscal activity classifiation and energy expenditure estimation. Some classical distance measures for signal processing and pattern recognition methods were understudied for this purpose. Briefly, the proposed method converts raw acceleration data into relevant spectral distances parameters.
We propose “dist-colorimetrics” (published in ICABME 2016), a methodology that aims to represent and classify ambulatory activities, based on the spectral distances measures. A 2D colored illustration of these parameters (Fig. 1) provides efficient visual representation as to the similarity and the variation among activities.
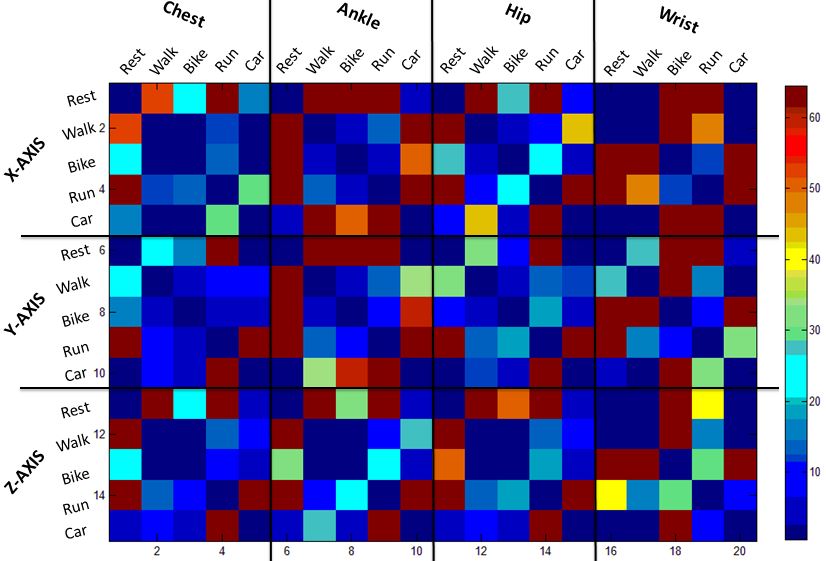
Fig.1 Color scaled 2D image resulting from the distances calculation by pairs of two distinct activities.
For a further validation in terms of performance recognition, the ‘dist-colorimetrics’ model was trained and tested by implementing three classification techniques, namely the Naive Bayes, the K-nearest neighbors and the decision tree. The results obtained proved a significant performance in predicting activities: more than 95% the rate of correctly classfied activities, obtained from 3 different algorithms (Table 1). The KNN classifier achieved the best average accuracy 98.12% (Table 2).
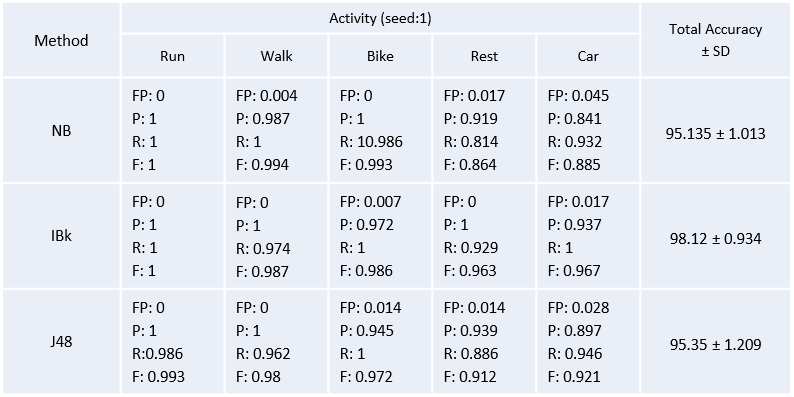
Table 1. False Poisitive (FP), Precision (P), Recall (R) and F-measure (F) Results for three classifiers and their corresponding average accuracy
Prospects for Future Works
After the activity recongition phase described above (submitted to the Biomedical Signal processing and Control Journal), the main issue is now to evaluate the energy expenditure. We already proceed to a litterature revue and design a new and original framework, compared to the litterature, to evaluate accurately the energy expenditure taking into account the ventilation rather than the resparatory rate as described in the litterature.