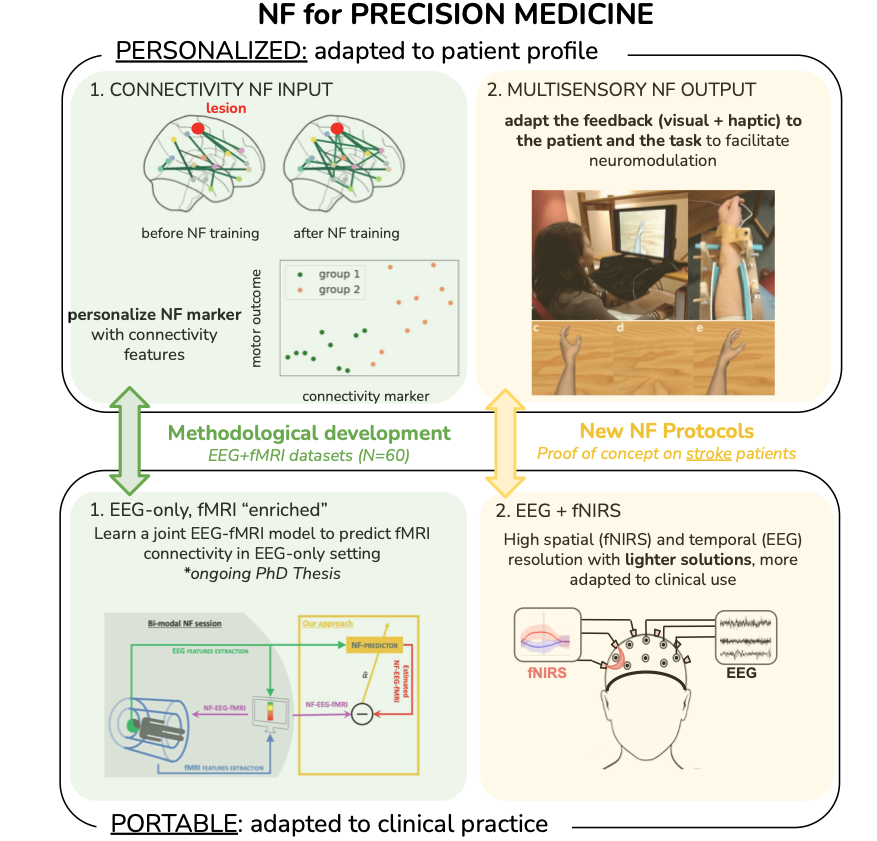
New ! Talk at the CominLabs days (18/11/2024) : slides
New ! We organized a symposium at the rtFin conference, in November 2024 in Heidelberg (Germany): “Multi-modal neurofeedback methods for post-stroke rehabilitation“. Part of the PEPERONI team presented their work :
- P.Maurel : “Simultaneous EEG-fMRI neurofeedback for post-stroke motor rehabilitation“, slides
- C.Muller : “Evaluation of multimodal EEG-fNIRS neurofeedback for motor imagery“, slides
- F. Le Jeune : “Introducing the Use of Thermal Neurofeedback“, slides
WP 1: Personalized Neurofeedback for improved neuro-rehabilitation
To improve the chances of success of NF neurorehabilitation, it is often necessary to be able to adapt the protocol to each patient. In stroke, for example, the position and nature of the lesions can change the optimal NF training strategy. We propose to improve the neuro-rehabilitation process, by addressing both sides of the neurofeedback pipeline, described in figure 2 : 1) the neurofeedback input (i.e. the brain patterns targeted by the neurofeedback training), and 2) the neurofeedback output (i.e. the feedback and sensory stimulations sent out to the user). These two research directions aim at personalizing the NF protocol by integrating connectivity information in the computation of the NF input and varying the nature of the NF stimuli
WP1.a: Adapting Neurofeedback “Input” Using Connectivity → Alix Lamouroux (PhD)
- Provide a tool to the community to process and analyse stroke data (under review in : Journal of Open Source Software)
- Benchmarking preprocessing pipelines for rs-fMRI data in stroke patients: the impact on functional connectivity and behavioral prediction
- Analysis of connectivity changes due to neurofeedback : a study on stroke patients.
WP1.b: Adapting Neurofeedback “Output” Using Multisensory Stimulations → François Le Jeune (post-doc)
- YUWIN – Stroke (earlY Upper Limb Rehabilitation WIth EEG-Neurofeedback After Stroke) : new study on 44 stroke patients. Investigate visuohaptic neurofeedback in early stage sensorimotor rehabilitation on clinical recovery scores and neuromodulation
- Insights on Thermal Neurofeedback for Brain-Computer Interfaces User Training : evaluates feasibility and effecRveness of thermal and visual feedback in EEG-based MI-BCI training.
WP 2: Towards a portable Neurofeedback
Simultaneous EEG-fMRI provides more specific, more efficient NF [7]. However the use of the MRI scanner is more costly and less comfortable for patients as compared to EEG-only NF sessions. In this project, we will investigate two research axes increasing the portability of our NF systems. On the one hand, the design of machine learning methods to improve EEG-only sessions with fMRI information. On the other hand, the introduction of the fNIRS modality, which has similarities with fMRI but whose acquisition system is much lighter. Our final goal is not to completely avoid MRI, this modality being crucial to provide good connectivity information for example, but rather to be able to use one or two EEG-MRI sessions to improve the efficiency of the NF protocol during non-MRI sessions (EEG alone or EEG-fNIRS).
WP2.a: Enhancing EEG-only NF Sessions by predicting fMRI scores → Caroline Pinte (PhD, not funded by CominLabs)
- Machine learning for bi-modal EEG-fMRI neurofeedback: EEG electrodes localization and fMRI NF scores prediction
WP2.b: Multimodal EEG-fNIRS Neurofeedback → Camille Muller (post-doc)
- implementation of a real-time acquisition system and processing chain for bimodal neurofeedback
- first study on 30 healthy subject (ongoing)