![]() |
CAPNEONATES (2011-2013) Analysis of structural MR and DTI in neonates |
Principal Investigators :
- Pierre Fillard and Bertrand Thirion, PARIETAL project team,Inria Saclay Ile de France
- Natasha Lepore, University of Southern California and Children’s hospital of Los Angeles
Research objectives:
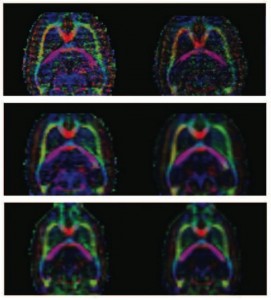
Improvement in FA color maps from our method, axial view of one slice. Rows from top to bottom: resolutions of 0:2mm3, 0:3mm3 and 0:4mm3. Left column: original acquisitions with the best CNR in each of the resolutions. Right col: Averaged subject with improved CNR.
Prematurity induced neurological problems are rapidly becoming one of the leading public health issues of this century. CAPNEONATES aimed to determine group differences in brain anatomy between normal newborns and premature babies at term equivalent age using MRI. The team’s goal was to develop a set of image processing and statistical analysis tools for the modeling and analysis of structural MR and DTI in neonates, and to compare the considered premature and control groups.
Scientific achievements:
The team designed a new tractography method to analyze term born and premature neonates.
- This method was adapted to perform reproducible tractography in rats using high spatial resolution at 7T.
- The team benchmarked some statistical analysis tools to enhance the detection of differences across populations..
Publications and Awards:
- 1 Journal article & 3 Conference papers.
Selected publication:
N. Lepore and F. Yepes, Y. Lao, A. Panigrahy, R. Ceschin, S. Ravichandran, M.D. Nelson, P. Fillard, Template-Based Tractography for Clinical Neonatal Diffusion Imaging Data, SPIE Medical Imaging, San Diego, California, USA, February 4-9 (2012).
Follow up:
CAPNEONATES generated other project ideas. In particular, the team has been working on a new machine learning method for the detection of Alzheimer’s disease in the clinic, which relies on the selection of discriminative features and a multivariate classification machine. The selection of a sparse set of features is particularly important to ease the clinical interpretation of the discriminative model. This development has been made relatively easy through the scikit learn library, contributed by PARIETAL.