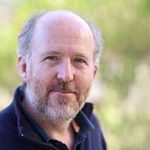
©A.Macarri-Université Côte d’Azur
Xavier Pennec, Inria research director at the Sophia Antipolis – Méditerranée centre in the EPIONE team, has just received the European Research Council (ERC) grant in the Advanced category for the G-Statistics project “Foundations of Geometric Statistics and Their Application in the Life Sciences”.
ERC Advanced Grants are awarded under the “Excellent Science” pillar of the European Union’s Horizon 2020 for research and innovation program and are attributed to senior researchers who are recognized as leaders in their field and who propose a research project that significantly pushes back the current frontiers of science.
Xavier Pennec is also the co-leader of the Inria@SiliconValley associate team GeomStats with Stanford University (Susan Holmes) on “Geometric Statistics in Computational Anatomy: Non-linear Subspace Learning Beyond the Riemannian Structure” (see last question in interview below).
Meeting with the new laureate who presents us his research and his project.
Can you tell us about your background before joining Inria?
After a baccalaureat in Mathematics and Technology and technical preparatory classes, I joined the Ecole Polytechnique in 1989.
I had already been programming for a long time, but I discovered the scientific side of computer science, especially with several teachers from Inria. This is what prompted me to choose to continue my research with a master and a thesis in computer science. I then did a post-doc at MIT in the artificial intelligence laboratory, before joining Inria as a researcher in 1998.
What is your field of research at Inria?
I am interested in shape analysis, and in the variability of human organs in particular, a field called computational anatomy . The mathematical problems that these morphological statistics raise are particularly interesting because one cannot add or subtract shapes. That is why we must reinvent statistical methods to work in these non-linear spaces. The potential applications in medicine are numerous because shape statistics enables the encoding of a priori knowledge on normal or abnormal anatomy.
There are also many other applications of statistics on geometric objects that I am interested in, specially in the life sciences. In fact, I believe that invariance properties (thus geometry) are among the most important priors to guide the statistical estimation towards more meaningful results in the small data regime. In the era of big data, this may seem strange to focus on small data samples, but big data actually tend to concentrate in certain areas of the parameter spaces where usually a lot is already known, while rare events are much more difficult to model and to predict. This is where science still has something to bring.
What is “G-Statistics” your project selected by the ERC?
G-statistics aims at exploring the consequences of the non-linearity of data spaces on the statistical estimation through geometry. We already know how to estimate the location (mean, median) and the concentration (covariance) of a random variable in a Riemannian manifold, or to perform simple statistical tests. There are also results for some classes of less smooth spaces, for instance length spaces of non-positive curvature. One of the objectives of geometric statistics is to unify these methods and to extend them to other non-Riemannian geometric structures. We want to include more complex spaces with singularities and changes of dimension, in particular affine connection, quotients or stratified spaces. These geometric structures appear in practical life sciences applications, as for example diffeomorphisms (invertible transformations of space) acting on images used in the registration of medical images, phylogenetic trees or shape spaces.
One of the key points I want to focus on is the impact of curvature, singularities and stratifications on the quality of the statistical estimation. This is especially important in the non-asymptotic regime because the number of data is always finite in practice. For example, curvature influences the concentration of an estimate and its gradient can induce a bias. When the data are sufficiently concentrated with respect to the curvature, these changes with respect to Euclidean statistics are not necessarily very important, but when one approaches a singularity, the curvature can become infinite and its impact becomes drastic.
A second aspect concerns data dimension reduction. It is often assumed that high dimensional data actually live on a small dimensional manifold (the manifold hypothesis). However, this assumption is often wrong because the optimal dimension depends on the scale at which the data are approximated and stratifications may appear. I think it is more interesting to construct a sequence of nested subspaces of increasing dimension which progressively approaches the data better and better, and to choose a posteriori the dimension, if necessary. The natural geometric notion that encodes this structure is that of flag manifolds for linear subspaces. I recently showed that Principal Component Analysis (PCA), which is ubiquitous in applied statistics, could be reformulated as an optimization on this flag manifold. The principle can also be extended to manifolds with more complex non-linear subspaces.
Finally, a third objective is to demonstrate the efficiency of these methods on selected applications in the life sciences field. Studying the variability of anatomical shapes using medical images is of course an application of choice for this, but other areas will also be considered.
Figure 1: Hessian IndexThe affine subspace generated by 3 reference points on the sphere of high dimension is a subsphere of dimension 2. The signature of the Hessian matrix of the weighted distance to references points (in black, their antipodal point in red) decomposes this 2-sphere in cells. The locus of local minima (Karcher Barycentric Subspace) in brown has a complex geometry that does not cover the whole sphere and can even be disconnected.
Figure 2 : shape of Dinausor foot-tracksResult of several barycentric subspace decomposition algorithms on the shape of Dinausor foot-tracks of Mount Tom with a L2 (left), L1 (center) and close to L0 (right) geodesic distance. |
Why did you choose these topics?
With medical imaging, I have worked since my thesis at the intersection of applications in medicine, computer science and several fields in mathematics, including geometry and statistics. For more than 15 years, I have been developing at Inria within the Project-team Epidaure, then Asclepios and now Epione, some medical image registration and morphometric methods that have allowed me to perceive the limits of current methods. For example, to go further in modeling complex shapes, it is necessary to consider changes of topology. Such a change corresponds to a singularity in the shape space with a stratification. But the behaviour of statistical estimation is very poorly known under such conditions. For example, colleagues have recently discovered that the mean is attracted towards the singularity under certain conditions (sticky mean), whereas we have shown with the recent theses of Nina Miolane and Loic Devillier that it can be repulsive under other conditions. It is therefore necessary to better understand the interaction of geometry with statistical estimation in order to discover approximate invariances (empirical laws) in life science data that are highly variable and very noisy. This is what led me to focus on the more fundamental aspects.
This prestigious grant is above all an extraordinary recognition by the scientific community for the field of geometric statistics as a whole and of the quality of research at Inria.
What does this grant mean to you?
The selection rate of ERC grants is such that many excellent projects are not selected, despite a peer-review selection system that seems particularly fair to me. This prestigious scholarship is therefore above all an extraordinary recognition by the scientific community. Beyond my work, I think this is a recognition for the field of geometric statistics as a whole and of the quality of research at Inria.
More practically, the grant also represents an extraordinary freedom in my research. Most current sources of funding for research require the justification of upstream theoretical research with short-term applications. Thanks to this grant, I have the possibility to devote myself entirely to science on fundamental theoretical subjects without having to constantly justify them. I think it is important to produce knowledge independently of its use if we want to induce conceptual or technological breakthroughs. Of course, I will illustrate my theoretical developments with applications that will highlight the interest of the methodology. But it is the crosspoint of this scientific knowledge with societal needs that might provide a posteriori the trigger for innovation. Not having to worry about it a priori represents a real freedom for research.
How do you plan to use this funding?
The ERC grant will allow me to recruit PhD students and young researchers to work on the above-mentioned subjects. I also plan to organize seminars to invite researchers in this field and workshops to share progresses during the project.
Are there other research tracks you would like to explore in the future?
Yes, of course. A better understanding of the interaction between geometry and statistics could help explaining the unreasonable effectiveness of current machine learning methods, and a contrario to understand their limitations. I am also interested in quantum information because it is based on deep geometric methods. Many other fields present applications at the crossroads of statistics and geometry.
But I have already a busy research agenda for the next 5 years with the G-statistics project!
Xavier, you are also the co-leader of the Inria@SiliconValley Geomstats associate team with Stanford University and USC. Can you tell us about this collaboration?
The associated team GeomStats with Susan Holmes’ lab is focusing on some specific problems in geometric statistics and their applications in computational anatomy where both teams have complementary knowledge. Susan Holmes has a deep expertise on statistics on metric spaces and their applications in biology, while I have a background in smooth differential geometry with applications in the medical domain. The effort in bridging the gap between our competencies was definitively instrumental in some of the ideas that I put forward in the ERC G-Statistics project.
Coming back to the subjects of the associated team GeomStats, we have investigated, in particular with the PhD of Nina Miolane, the statistical consistency of the estimation in quotient spaces. This problem naturally appears in neuroimaging when computing the template image to compute anatomical or functional image biomarkers. Understanding the quotient structure of brain images under the action of deformations allowed us to understand that the topological changes of the intensity isolevels induce a stratification of the image space. One of the ideas that we are now pursuing with Nina’s post-doc is to exploit this stratified structure to encode the variability of the brain anatomy in a multi scale tree-like structure where we can guarantee the consistency of the statistical estimation. This may provide more realistic templates for neuroimaging studies. We also plan to study other subjects like subspace learning in non-linear spaces with applications that are complementary to the one of my ERC project.
Five key dates in Xavier Pennec’s carrier
Xavier is also teaching at ENS Cachan, Ecole Centrale, and at Université Côte d’Azur |
Source: https://www.inria.fr/en/centre/sophia/news/erc-advanced-grant-for-xavier-pennec (edited)
Related links